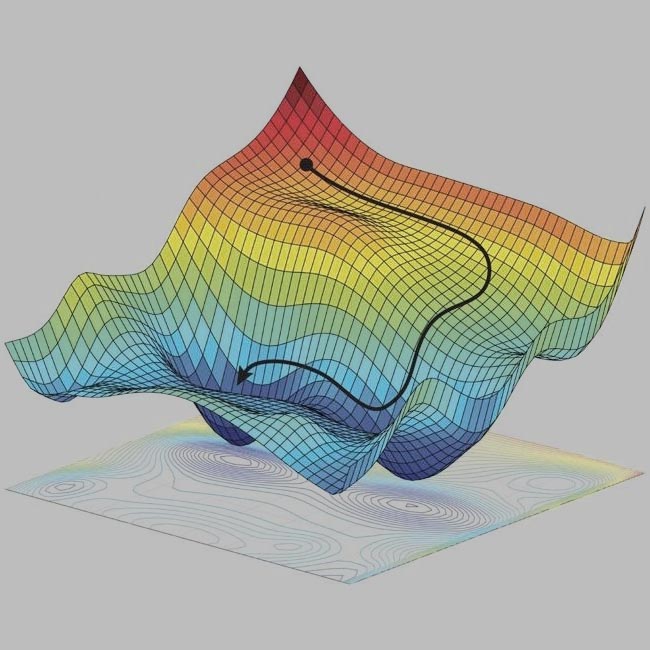
Optimization in Deep Learning
Optimizers are essential components of machine learning algorithms, responsible for adjusting the parameters of a model to minimize the loss function. A loss function measures how well the model’s predictions match the true values, and the optimizer helps find the parameters (weights) that minimize this loss. Essentially, the optimizer guides the model to find the optimal set of weights that result in the best performance. In this blog post, we will dive into the basics of some popular optimizers used in machine learning: Stochastic Gradient Descent (SGD), SGD with Momentum, and Adam. We will also visualize how each of these optimizers performs in finding the minimum of a given loss curve. ...